Introduction
In the rapidly evolving landscape of AI, the need for systems capable of generating meaningful, contextually accurate content is paramount. Retrieval-Augmented Generation (RAG) stands at the intersection of retrieval systems and generative models, enabling better reasoning and decision-making by accessing vast external data repositories. This article explores how reasoning in RAG enhances AI performance and drives impactful real-world applications.
The Basics of RAG
At its core, RAG leverages two key components:
- Retrieval Mechanism: This component searches external databases or knowledge sources for relevant information based on the input.
- Generative Model: A language model that processes the retrieved data and generates coherent, contextually relevant content.
The fusion of these components allows RAG systems to surpass traditional models, which are limited to static training data. With RAG, models gain dynamic access to real-time, updated information, improving the accuracy and relevance of their output.
The Role of Reasoning in RAG
Reasoning is what sets advanced RAG systems apart from simple data retrieval. When combined with a powerful reasoning layer, RAG can:
- Contextualize information: The system can assess the relevance of retrieved data and discard irrelevant or outdated information.
- Chain multiple facts: RAG uses logical reasoning to connect different data points, creating coherent and comprehensive responses.
- Improve decision-making: By leveraging both external knowledge and reasoning capabilities, RAG can offer suggestions or conclusions based on a nuanced understanding of the problem at hand.
How Reasoning in RAG Works
Reasoning in a RAG system is typically built on the following processes:
- Semantic Understanding: The AI can understand the relationships between concepts by analyzing their vector representations. In systems like Aime’s RAG, the XDB database plays a crucial role by managing both Graph Objects and vector data, allowing the AI to “reason” based on concept proximity and relational data.
- Iterative Search and Generation: The AI retrieves additional data as needed, refines its search based on intermediate results, and generates progressively more refined outputs.
- Dynamic Data Integration: The reasoning process allows the AI to integrate and evaluate new information, creating a feedback loop that refines the final output.
Aime’s RAG Solution: Bridging Reasoning and XDB
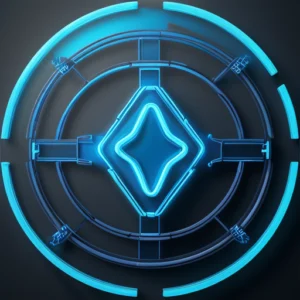
Aime’s RAG solution, built on its powerful XDB database, demonstrates the seamless integration of reasoning and retrieval. XDB manages graph and vector data in real time, allowing RAG to extract not only relevant information but also make connections between disparate data points. This leads to more insightful and semantically rich results.
By incorporating reasoning capabilities, Aime’s RAG solution enhances the system’s ability to generate contextually aware content that aligns with the user’s needs. This integration showcases the benefits of connecting reasoning directly to the underlying data infrastructure, allowing systems to “think” while generating.
Real-World Applications of Reasoning in RAG
Reasoning in RAG isn’t just theoretical; it’s impacting several industries, including:
- Healthcare: Assisting doctors by retrieving and reasoning over medical records to suggest potential diagnoses.
- Customer Support: Handling complex queries by reasoning over historical conversations and FAQs to deliver more accurate answers.
- Legal Research: Retrieving relevant case law and reasoning through legal precedents to assist lawyers in building strong arguments.
Conclusion
As AI systems continue to evolve, the need for advanced reasoning in RAG will grow. By combining retrieval with dynamic, logic-based generation, RAG systems can move beyond basic information delivery, offering profound insights and meaningful interactions. Aime’s RAG solution, built on XDB, exemplifies this powerful combination, pushing the boundaries of what AI can achieve in real-world applications.